Materials discovery: quantum computing and deep learning, complementary technologies
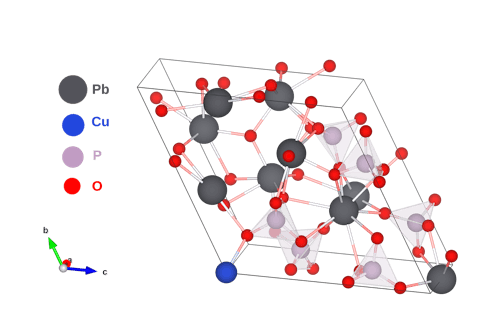
Yesterday Google Deepmind announced the exciting discovery of 2.2 million new crystals through the use of their new ‘Graph Networks for Materials Exploration’ (GNoME) deep learning tool. This is an exciting development in a field where the discovery of new materials is often hindered by the time required for complex simulations to yield results.
Google Deepmind’s approach combines novel methods to generate new candidate structures and state-of-the-art graph neural networks to model the materials’ properties. The final step in their approach exploits Density Functional Theory (DFT), a well-established method to describe materials’ properties, to compute the energy and verify the stability of the new structures, which are then used as new training datasets for the tool.
Whilst widely used, DFT has well-known weaknesses in its ability to accurately predict the electronic structure (and subsequent material behaviour) for many materials where quantum electron correlation effects play a major role. Even though this can be partly mitigated by the use of sophisticated functionals such as rSCAN2 (used in GNoME), DFT predictions typically need to be verified and improved through more complex (and computationally intensive) methods or the ultimate arbiter, experiment.
Moreover,whilst a thermodynamically stable structure provides a well-defined computational target, materials discovery often requires the identification of metastable phases which can work under specific conditions. This is an important consideration, especially given the fact that most nanoparticles (including catalysts and battery materials) are metastable by definition (with the stable state being a bulk system). Finally, arguably the ultimate step in the discovery process for advanced materials is the ability to relate a material to a use, or function, which effectively converts the new material into something with real-world applications. Short of (lengthy and expensive) experimental testing, current methods to predict a materials’ function rely on approximate trends being captured correctly.
An opportunity to transform this paradigm, though, comes from quantum computing, where we know that accurate simulation of materials’ properties is indeed possible. By exploiting the quantum nature of the hardware itself, even with relatively small quantum computers it is possible to run simulations at a level of precision that is beyond classical computers’ ability to compute. Some of the algorithms developed by Phasecraft in recent months have been addressing this specific challenge and could provide improved data and predictions. These algorithms are still beyond the capabilities of existing quantum hardware, but with the current expected rates of hardware development, within a few years they will be able to enhance tools such as GNoMe, enabling a further and potentially revolutionary advance in the discovery of new functional materials.
By Glenn Jones, Principal Quantum Scientist, Phasecraft