Startup Aims Algorithms at Quantum Error Correction
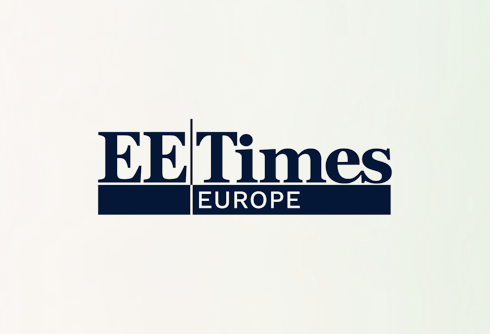
Quantum computers can perform complex computations in a fraction of the time required by classical computing architectures, promising benefits in industries such as drug discovery, financial modeling and cryptography, but quantum error rates must be reduced before that goal can be broadly realized. London-based startup Phasecraft is creating and implementing algorithms to lower the error rate of quantum computers and is working with several quantum hardware providers to advance the pace of development.
In an interview with EE Times Europe, Ashely Montanaro, a Phasecraft co-founder and director, discussed the company’ history, mission and projects. Montanaro has worked in the field for more than 15 years, specializing in quantum algorithms and quantum computational complexity, and has published over 50 papers on the topic. He holds a Ph.D. in quantum computing from the University of Bristol and is a professor of quantum computation there.
Foundation and mission
Montanaro co-founded Phasecraft in 2018 with fellow quantum computing scientists Toby Cubitt and John Morton. Like Montanaro, Cubitt has spent many years developing quantum algorithms, and both have received awards for their work. Morton has 20 years of experience in quantum computer R&D and is the director of the UCL Quantum Science and Technology Institute.
Collaborating with quantum hardware providers including Google Quantum AI, IBM Quantum and Rigetti, Phasecraft aims to reduce the error rate of quantum computers by leveraging the unique properties of quantum mechanics. The company has developed a range of strategies to achieve this goal, including new developments in quantum circuits, error-correction codes and algorithms.
A detail of the IBM System One quantum computer, showcased at CES 2020 in Las Vegas.
For example, Phasecraft has developed a quantum error-correction code that can increase the error tolerance of a quantum computer by several orders of magnitude. The code applies advanced techniques from quantum information theory to detect and correct errors that arise during computation.
According to Montanaro, one of the most relevant applications of quantum computing is the simulation of quantum mechanical systems, a challenging task even for classical high-performance computers (HPCs). Typical use cases include the simulation of batteries for electric vehicles and solar cells.
Because quantum computers can run complex simulations of materials and chemical compounds, they could be used to find new EV battery materials to increase the vehicles’ driving range on a charge or reduce their weight, size and cost. One of the main challenges in developing better batteries is understanding the complex interactions among the various materials used in the battery. Those interactions occur at the atomic and molecular levels and can be difficult to predict using classical methods, but quantum computing can potentially model the materials’ behavior and interactions in greater detail. That could lead to the development of more efficient and longer-lasting batteries by identifying optimal materials and configurations for energy storage.
Furthermore, quantum computing can help design algorithms for the efficient charging and discharging of batteries at the molecular scale. Those algorithms could potentially lead to more effective and faster battery charging and longer battery lifetimes.
Similarly, quantum computing has the potential to advance the development of more efficient solar cells by enabling researchers to model the complex behavior of materials more accurately at the quantum level. Benefits of quantum-based simulation include:
- New materials discovery. Quantum computing can simulate materials’ electronic and optical properties, making it easier to find new materials that would be more effective at converting sunlight into electricity.
- Chemistry optimization. Quantum computing can accurately simulate the behaviors of atoms and molecules, allowing researchers to develop more efficient chemical processes for producing solar cells.
- Performance modeling. Quantum computing can be used to model the performance of solar cells under different conditions, such as varying light intensity and temperature. By understanding how solar cells behave in different situations, researchers can develop better designs and materials for solar cells that can operate effectively under a wider range of environmental conditions.
Scalability, both at the hardware and software levels, is an essential requirement to address the evolution of quantum computing. Phasecraft implements scalability in its algorithms in several ways, including error correction, which is essential to quantum computing because quantum systems are inherently noisy. Phasecraft says it uses error-correction techniques that enable high accuracy even in the presence of errors.
A further method for implementing scalability is the hybrid quantum-classical approach used in Phasecraft’s algorithms. By using classical computers for pre-processing and post-processing steps and quantum computers for the core computational tasks, Phasecraft can achieve speedups without requiring a fully fault-tolerant quantum computer, according to Montanaro. Moreover, Phasecraft’s algorithms are designed to be modular, meaning they can be easily scaled up or down depending on the problem’s size. This modularity makes it possible to implement Phasecraft’s algorithms on different hardware platforms with varying numbers of qubits.
Another key area of Phasecraft’s research is the study of quantum phase transitions, which occur when a quantum system moves from one state to another in response to changes in external conditions such as temperature or pressure. Understanding quantum phase transitions is crucial to the development of algorithms that can solve complex problems on quantum computers.
Phasecraft has developed a range of techniques that can be used to simulate quantum phase transitions, allowing researchers to explore its properties in detail. For example, the company leverages the Variational Quantum Eigensolver (VQE), a quantum algorithm used to solve the ground state energy of a given Hamiltonian by using a quantum computer. It is a hybrid algorithm that combines elements of both classical and quantum computation.
Making the most of NISQ computers
An advantage of Phasecraft’s algorithms is their ability to run on near-term intermediate-scale (NISQ) computers, said Montanaro. NISQ represents a class of currently available quantum computers whose quantum operations and measurements are subject to noise and errors, making it difficult to obtain accurate and reliable results with many qubits. These quantum computers typically have a limited number of qubits, ranging from tens to a few hundred. Despite their limitations, NISQ computers are valuable for studying and exploring the potential of quantum computing.
As NISQ computers are currently the most advanced quantum machines, developing software and algorithms that can effectively run on them is important for the advancement of quantum technologies. Phasecraft is addressing the challenges of NISQ computing by developing algorithms and techniques (such as error correction) that maximize the use of the limited resources available. Developing software and algorithms for NISQ machines may help build a foundation for scalable quantum computers in the future.