Peptide conformational sampling using the Quantum Approximate Optimization Algorithm
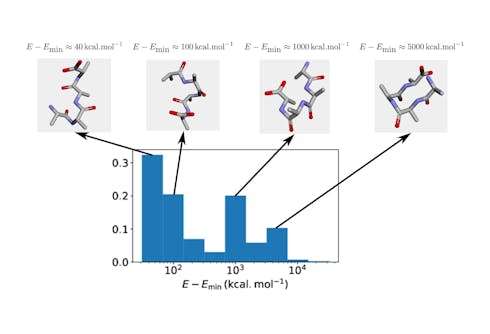
Phasecraft researchers Sami Boulebnane and Ashley Montanaro recently published a paper in npj Quantum Information on applying quantum algorithms to protein folding, resulting from a collaboration with a leading pharmaceutical company.
Predicting the structure of proteins — also known as the protein folding problem — is a major research frontier in computational biology, with potential groundbreaking applications in the understanding of certain diseases and for the pharmaceutical industry. Namely, understanding proteins on a computer could save costly and time-consuming lab experiments, allowing us to screen more drug candidates faster, for instance.
After decades of incremental progress, the field experienced a revolution in 2021 with the release of AlphaFold, a deep-learning model capable of predicting the shape of many proteins with unprecedented accuracy. So is it fair to say that “protein folding is solved”? Not quite!
First, at the fundamental level, the model performs less well on certain “exotic,” yet still practically relevant, protein structures. Next, at the practical level, the underlying model is very costly to train and maintain. These outstanding issues justify continued research in the field. “Alternative” computational paradigms such as quantum computing offers a great deal of potential as one such novel direction.
Broadly speaking, quantum computing may help with protein folding in two very different ways. The first is the “quantum simulation” approach, allowing quantum computing to better simulate the physical and chemical processes happening at the microscopic scale in the protein, for which quantum effects must be accounted. The other way is the “combinatorial” approach, enabling faster search among the astronomical number of potential protein structures.
In this collaboration, we investigated the performance of a well-known quantum algorithm, the Quantum Approximate Optimization Algorithm (QAOA), in addressing the combinatorial formulation of protein folding.
Due to the high resource requirements of the algorithm when applied to this specific problem, we had to rely on classical simulations rather than running on real quantum hardware. Besides, due to the intrinsically limited ability of classical computers at simulating quantum ones, we had to limit ourselves to a very small protein that included 4 amino acids with a focus on the backbone atoms.
With this necessary restriction on the size and complexity of the protein, the first step of the study voluntarily simplified the problem even further: rather than sampling the most energetically favourable protein structure, we required merely sampling a “valid” one. More specifically, a valid structure is one where two atoms do not occupy the same site. As evident as this requirement may sound, a solution produced by QAOA is not guaranteed to satisfy it. Importantly, one cannot just assume there is a good chance for the structure to be valid, since such solutions form an exponentially small part of the exhaustive set of configurations. Fortunately, this first step of the work empirically established that QAOA could be trained to produce correct configurations with relatively high probability.
Encouraged by these initial results, we then considered a slightly more realistic version of protein folding, with the goal of obtaining a low-energy conformation among valid ones. Surprisingly, adding this extra constraint significantly degraded the performance of QAOA: while it was still possible to sample mostly valid structures, the energy of these structures struggled to converge to the optimum despite many iterations of the algorithm.
A potential interpretation of the results is as follows. On the one hand, the requirement of producing a valid conformation can be seen as a constraint satisfaction problem: namely, the aim is to satisfy a set of constraints, where in this case each atom of the protein is constrained not to clash with the others. On the other hand, finding a low-energy conformation among valid ones is a matter of optimizing a real-valued function. Since QAOA did well in the former setting but proved less compelling in the latter, our results suggest the algorithm should be used to solve discrete constraint satisfaction problems rather than continuous optimization ones.
We hope our work will provide useful methodological elements to the practitioner for critically benchmarking QAOA and other quantum optimization algorithms on further real-world problems.
Sami Boulebnane
The paper was coauthored by:
- Sami Boulebnane, University College London and Phasecraft Ltd
- Xavier Lucas, Roche Pharmaceutical Research and Early Development
- Agnes Meyder, Roche Pharmaceutical Research and Early Development
- Stanislaw Adaszewski, Roche Pharmaceutical Research and Early Development
- Ashley Montanaro, Phasecraft Ltd and University of Bristol
You can read the paper here.